The Student Presentation Chair of the ASME Internal Combustion Engine Division invites you to submit your undergraduate research in internal combustion engines. The top two entries will be selected to be present at the ASME ICE Forward 2024 Conference which will be an in-person event. The two students who led the winning entries will receive free conference registration for the conference along with paid travel and lodging expenses for the conference up to $1,500 for the winners.
Consider applying if you:
- Work (or have worked) in a laboratory that does internal combustion engine research
- worked on modeling and simulation related to engine systems or emissions systems
- worked on fuels, fuel injection, sprays or carbon management
- worked on the engine of a collegiate race team or your own car
- have innovative ideas for next generation advanced engines, or alternative fuels
- are an internal combustion engine enthusiast and would like to deliver a presentation on the subject
The winning two students will deliver their presentations to a group of leading experts in the internal combustion engine field at the ASME ICE Forward 2024 Conference. This welcoming environment is a great opportunity for students currently involved in research that are considering pursuing a career or graduate school in the Internal Combustion Engine field. Many of the past winners have made connections during the conference lead to recruitment for career and graduate school opportunities. For senior undergraduate students who may have already accepted a full-time position or begun graduate school by the time the conference is held, it is also a great way to be introduced directly to a large portion of the engine research community that you may be a part of for many years to come.
Additionally, as a conference attendee, you'll get to attend other researcher's presentations and network with people working in this exciting and important field. Applicants not selected as winners will be invited to present their work at a student poster session to be held during the conference.
To be considered, submit the following to the Student Presentation Chair by July 19th, 2024:
- an abstract/summary of your work in pdf format (2-pages max),
- 10-minute draft presentation slides as a pdf file,
- a one-page mentor/advisor letter of recommendation. The letter of recommendation must be from a professor or mentor working most closely with the student and must clearly identify the student’s role in the work.
Scoring Criteria
A selection committee will choose the top two presentations according to an established review process. In that process, submissions will be scored according to the following metrics:
You must be an ASME student member (with active membership) and had been an undergraduate student on the date of submission to be selected (if you will graduate in or around May 2024, you are still eligible). Presentation material must all be original. The work can be related to material contained in a technical paper submitted to the ASME ICE Forward 2024 Conference but should go beyond what is in the paper.
- technical strength,
- research novelty
- quality of project execution,
- degree of independent work
- presentation quality
Send your abstract, draft presentation, and letter of recommendation by July 19th to:
Dr. Noah Van Dam
Student Presentation Chair
Email: Noah_VanDam@uml.edu
2023 Winners
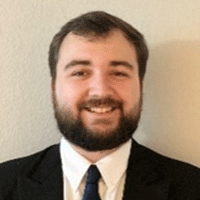
Nicholas Dillon
Georgia Southern University
Statesboro, GA
Presentation:Investigations of Low-Reactivity X-98 Ethanol in Reactivity Controlled Compression Ignition with High-Reactivity Jet-A for Performance and Emissions Improvement
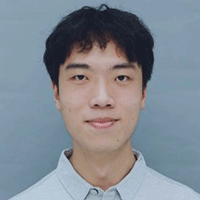
Ziming Zhou
University of Michigan–Shanghai Jiao Tong University Joint Institute
Shanghai, China
Presentation: Planar In-cylinder Flow Field Prediction based on Physics-inspired Automated Machine Learning Framework