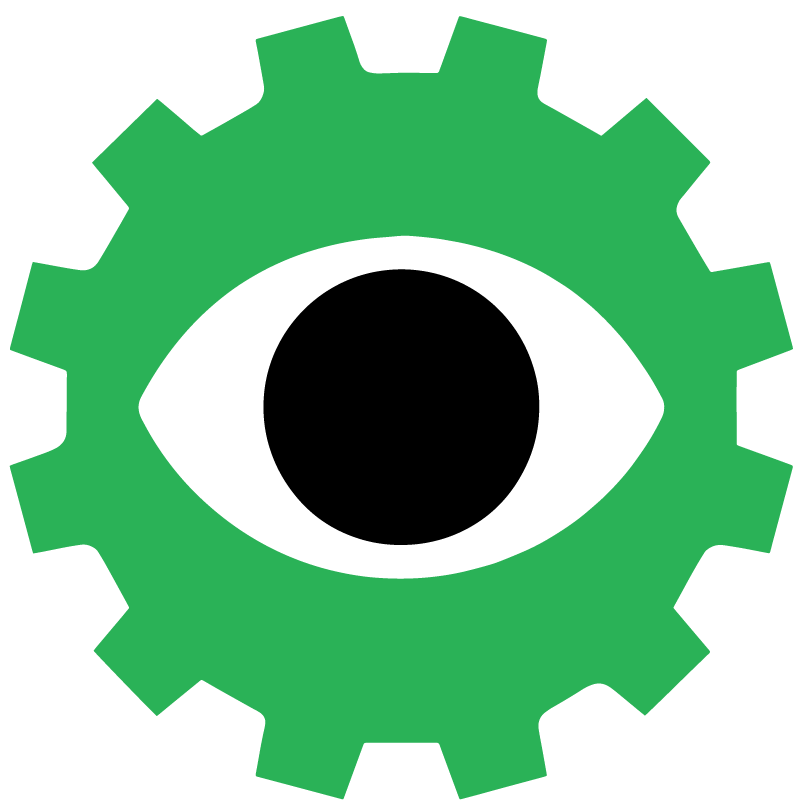
ASME 2024 Student Hackathon
Harnessing Artificial Intelligence to Enhance Mechanical Engineering
Background
The Computer & Information in Engineering (CIE) Division of the American Society of Mechanical Engineers (ASME) held past hackathon events at the IDETC/CIE 2020, 2021, 2022, 2023 Conferences. These hackathon events provide students and engineering practitioners with a unique opportunity to learn how data science and machine learning techniques can be leveraged to solve real-world engineering problems.
Given the previous resounding successes, the CIE Division will hold the ASME-CIE Hackathon again at the IDETC/CIE 2024 Conference in hybrid settings, both virtual and on-site, as a pre-conference event from Aug. 18-25, 2024.
JW Marriott, Washington, DC
August 16 – 24, 2024 virtually and August 25, 2024 in person
in conjunction with
ASME IDETC/CIE 2024 Conference (August 25 – 28, 2024 in person)
Sponsored by
ASME Computers & Information in Engineering Division (CIE)
Onshape
and
UES Inc.
There is a nominal registration fee waiver and a limited number of travel awards
Please fill in this application form and send it with your CV to binyangs@vt.edu to apply by August 12th. This will be a hybrid event, both virtual and on-site. If unable to attend in person, please email us at idetccie.seikm@gmail.com so we can connect with you virtually.
Participants are not limited to the number of problems chosen.
Important Dates
August 15, 2024, 11:59pm EDT: Registration Deadline
August 16, 2024, 12 – 3pm EDT: Virtual Hackathon Kick-off
August 25, 2024: Hybrid Hackathon Closing
August 25, 2024, 6am EDT: due for Hackathon Deliverables
August 25, 2024, 10:30am – 4:15 pm EDT: Final Presentations
August 25, 2024, 4:15 – 5:30pm EDT: Hackathon Judging
August 25, 2024, 5:30 - 6:30pm EDT: Closing Ceremony
* By registering for the Hackathon, you agree to allow your information to be shared with other registrants and volunteer leaders for the purpose of communicating event information and intra team communication.
Award Information
For each problem:
- First Prize: $1,400
- Second Prize: $700
- Third Prize: $350
Notes: Teams will be judged in each problem topic area, and awardees will be selected separately.
Eligibility
Undergraduate students, graduate students, postdocs, and non-students (e.g. professionals) are welcome to attend the Hackathon and experience the exciting competitions.
Problem Sets: The full dataset can be accessed via the GitHub webpage
Problem Statement 1
National Institute of Standards and Technology Technical; NIST
In the realm of additive manufacturing (AM), especially powder bed fusion, real-time monitoring plays a pivotal role in ensuring process integrity. Equipped with sophisticated in-situ sensors, modern AM machines, like those at the National Institute of Standards and Technology (NIST), generate vast datasets during each build, exemplified by our testbed's co-axial camera which captures up to 20,000 frames per second. Despite this advanced capability, data transfer limitations can lead to occasional image loss. For instance, discrepancies have been noted where the camera log records more images than are actually retrieved—sometimes with a shortfall of up to 50 frames per 200,000 captured. This hackathon challenge centers on developing innovative methods to accurately locate these missing frames within melt pool monitoring (MPM) image sequences. Participants are invited to tackle this issue in two parts: the first assumes missing images can be re-identified without their original sequence, while the second deals with scenarios where the missing frames are irretrievably lost. Solutions will be assessed based on accuracy, creativity, and clarity, with results evaluated after a week.
Problem Statement 2
UES-AFRL
The AFRL FactoryNet dataset, designed to enhance machine vision AI systems in the manufacturing sector, features a vast collection of images sourced from web scraping, machine shops, factories, and industry partners. This diversity presents significant challenges in scope definition, organization, and curation, particularly at its current stage—organizing and collecting human-generated labels. This hackathon problem challenges participants to navigate the complexities of multiple unstructured labels to extract the most value from the dataset. The task involves sanitizing and structuring these labels in a way that aids the development of image classification models. By consolidating freeform labels and demonstrating their utility through successful model training and validation, participants will highlight the dataset's strengths and areas for improvement. The goal is to produce structured, meaningful classes that enhance the dataset's applicability in AI-driven image recognition within manufacturing. Successful entries will clearly demonstrate the creation of effective classification models and the strategic organization of label data.
Hackathon Team and Presentation
- All participants must be registered by August 5, 2024 11:59 pm EDT. Everyone will be placed in a team up of 1-2 members. You may form a team based on your own preference. All implementations must be based on the original work.
- Each Hackathon team will continue their own meetings via their own chosen platform between 08/18/24 and 08/25/24.
- Each team needs to present their teamwork including the technical approach and submit the results to their own GitHub repository by the submission deadline.
- Team final presentation, results, and technical approaches will be evaluated based on a technical committee (separated with the Hackathon organizing committee).
Hackathon Organizers
Prof. Binyang Song (Virginia Tech) - Chair, binyangs@vt.edu
Dr. Anh Tran (Sandia National Laboratories), anhtran@sandia.gov
Prof. Zhenghui Sha (University of Texas – Austin), zsha@austin.utxas.edu
Prof. Hyunwoong Ko (Arizona State University), hyunwoong.ko@asu.edu
Dr. Zhuo Yang (Georgetown University, National Institute of Standards and Technologies), zy253@georgetown.edu
Dr. Laxmi Poudel (General Electric), Laxmi.Poudel@ge.com
Dr. Yan Lu (National Institute of Standards and Technologies), yan.lu@nist.gov